Ethical considerations in multilabel text classifications
The Our Community Innovation Lab have examined the CLASSIEfier algorithm to identify biases and attempt to address them.
All SmartyGrants users have access to the insights gleaned from CLASSIE – the social sector taxonomy developed by Our Community. And some of you may have heard of CLASSIEfier, a tool based on CLASSIE that automatically identifies the subject and beneficiaries of a grant application in SmartyGrants.
But how trustworthy is an automatic classification tool? What assurances do SmartyGrants users have that the tool is accurate? Our Community’s Innovation Lab data scientists set out to find out.
The results of their research are summarised in a white paper, Ethical Considerations in Multilabel Text Classifications.
Summary of our findings:
- Bias is difficult to remove entirely. Maintaining transparency and open communication with users about the possible gains and pitfalls of using auto-classification can be a useful tool in fixing bias.
- For the purposes of CLASSIEfier, a keyword-matching approach outperforms machine learning, neural networks and transformers because it allows for greater transparency and adaptability.
- More work is being done to improve the CLASSIEfier algorithm’s accuracy – stay tuned for updates. SmartyGrants users can play a role in this by continuing or adopting the use of CLASSIE when designing their grant programs. Learn more about CLASSIE here.
What is CLASSIEfier?
CLASSIEfier is an auto-classification algorithm developed by the Our Community Innovation Lab that uses a social sector taxonomy, CLASSIE, to classify grant applications and other social sector text.
Learn more about CLASSIE and CLASSIEfier.
Why is reducing or eliminating bias important?
Algorithms inherit the biases of the humans who create them; this is commonly accepted in the data science community. However, when those algorithms are driving decision-making at scale, and potentially influencing funding decisions, it is imperative that we strive to reduce bias as much as possible.
Let’s say your organisation puts out a call for applications for its annual grant round which funds projects that support Culturally and Linguistically Diverse (CALD) people and migrant families.
An applicant contacts you confused; even though they read the guidelines thoroughly and believed they met all of the criteria, their application was rejected.
You discover that the text classification algorithm your organisation employs has incorrectly classified the word ‘space’ – mentioned several times in their application in reference to building a shared community space for migrant mothers and their children – as belonging to the Aerospace engineering category. This label led to the application being deemed as ineligible for funding.
Text classification algorithms can struggle to differentiate context in language; humans, less so. After a phone conversation, your program manager reviews the application again and the organisation receives funding for their project.
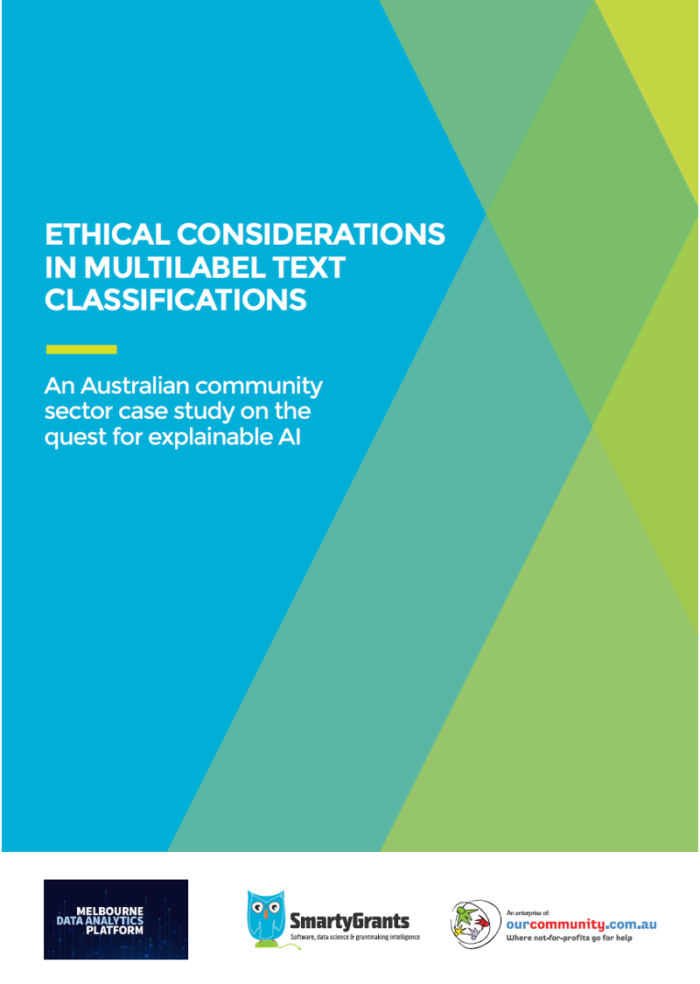
In summary
CLASSIEfier has measures in place to counter this kind of word bias, such as requiring word matches in different groups (topic and context) and incorporating an exclusion group of words. But there is still work to be done to improve the tool, with the white paper’s main recommendation being to maintain transparency and communication with users about the biases we notice and the decisions that have been made to address them.
Although best efforts are to make the algorithm as accurate as possible, the end user’s expectations will change depending on the program they are administering and areas of focus. For this reason, it is recommended that the CLASSIEfier algorithm (or any algorithm, for that matter) is not used without human oversight.
In the meantime, testing and feedback will continue to improve the algorithm’s accuracy.
That’s why we encourage all SmartyGrants users to use the CLASSIE taxonomy when building their application forms. By providing a bigger picture of where and to whom money is flowing, and to what use it is being put, CLASSIE will ultimately enable changes that will strengthen the social sector.
Read the full report for more on CLASSIEfier and how it will help your organisation streamline its processes and make for smarter, more evidence-driven practice.
This project was completed in partnership with Melbourne Data Analytics Platform (MDAP) at the University of Melbourne. Building on the success of this collaboration, MDAP is keen to continue to engage with the wider community.